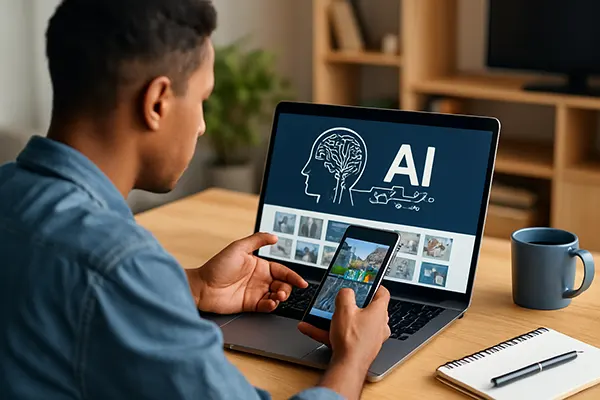
Microservices in the AI Era: Earning with Artificial Intelligence Microtasks
As artificial intelligence becomes an increasingly integral part of business operations and everyday life, a new economic opportunity has emerged — providing microservices to AI systems. These are not traditional software services but small, repeatable human contributions that help AI models learn, adapt, and function effectively. In February 2025, the demand for such microservices is higher than ever, offering freelancers, students, and digital workers a way to earn steadily by supporting AI’s growth and utility.
What Are AI Microservices and Why They Matter
Microservices in the context of AI are typically small-scale, human-driven tasks or contributions that assist machine learning algorithms in improving their accuracy, reliability, and human-likeness. These tasks range from labelling datasets to moderating AI-generated content, providing feedback on AI outputs, or performing rapid validation checks on translated text or summarised material.
As large language models and generative AI tools evolve, the volume of real-time data they require grows exponentially. However, not all data is usable “as is.” Human-in-the-loop systems allow AI to be corrected, fine-tuned, and enhanced through microservices that supplement automated processes. These small interventions help prevent bias, ensure linguistic accuracy, and enforce ethical standards.
Companies like OpenAI, Google, and Meta increasingly rely on microtask platforms — such as Amazon Mechanical Turk, Scale AI, and Remotasks — to outsource these bite-sized operations. This trend has turned into a substantial sector of the digital gig economy, providing paid work to contributors globally.
Examples of In-Demand AI Microservices
Content labelling remains one of the top microservices required by AI companies. These tasks involve classifying text, audio, or images into categories — for instance, tagging whether a social media post is sarcastic, offensive, or neutral. AI systems then learn to make similar judgements on their own.
Another growing field is AI training validation. Workers might be asked to compare two pieces of AI-generated content and indicate which is clearer, more human-like, or grammatically correct. This comparative feedback helps fine-tune AI decision-making models.
Audio transcription and text correction services are also in high demand. These require fluency in specific languages and can yield higher rates of pay. Such microservices are critical for enhancing speech recognition software and multilingual AI performance.
Platforms Where You Can Earn with AI Microservices
Several reliable platforms offer structured access to AI-related microtasks. Remotasks, operated by Scale AI, is one of the most active hubs. It provides varied tasks — from image annotation to lidar data processing — and includes training modules to help users qualify for more complex, better-paid assignments.
Amazon Mechanical Turk (MTurk) continues to be a classic go-to for microtaskers, especially in English-speaking markets. It features a wide range of tasks that support natural language processing models, such as entity recognition or sentiment analysis. However, MTurk may not be accessible in all regions, and registration can be selective.
Appen and Clickworker are also trusted platforms that frequently offer AI-related projects. These include voice recording tasks, user behaviour tracking, and search engine evaluation. Payments can vary based on task complexity, required language skills, and project timelines.
Important Considerations When Choosing a Platform
Before starting with any microtasking service, it’s important to verify its credibility. Check user reviews, payout history, and whether the platform operates transparently with clear instructions and fair compensation. Reliable platforms also offer dashboards that let workers track their earnings and progress.
Geographic limitations can affect accessibility to certain platforms or task categories. Some AI training projects require contributors from specific regions or with native-level fluency in less commonly used languages. This can be either a limitation or an opportunity depending on your background.
Finally, consider platforms that offer certification training or upskilling paths. The ability to complete advanced qualification tests often unlocks higher-paying microservices and long-term contracts, especially in fields like biomedical data annotation or AI ethics auditing.
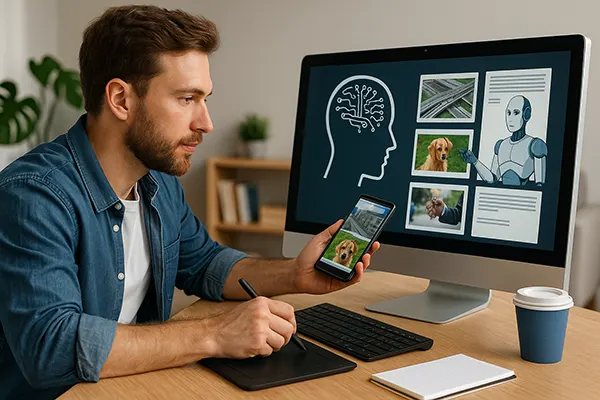
Monetisation Strategy and Realistic Earnings in 2025
In February 2025, experienced microtask workers can earn anywhere from $5 to $25 per hour depending on the complexity of tasks, speed, and platform conditions. Those involved in niche or technical services such as programming code evaluation or medical text review may earn more consistently at the higher end of this range.
To maximise income, contributors often register on multiple platforms and combine their schedules. Effective time management and high task approval ratings improve one’s visibility to task requesters, increasing job availability and rates. Some microtaskers also use browser automation tools or work within digital cooperatives to increase efficiency and share best practices.
Microservices can also become a stepping stone into more stable careers in AI-related roles. Many data annotators have transitioned to roles in prompt engineering, AI model testing, and digital research assistance. Platforms like Toloka and TranscribeMe now even list part-time project manager roles for top-rated contributors.
Skills and Tools That Increase Your Value
Fluency in English and other widely spoken languages remains a core asset. However, in 2025, knowledge of regional dialects, slang, or cultural references — especially for low-resource languages — gives workers a competitive edge in microservices involving localisation or emotion detection.
Experience with tools such as Labelbox, SuperAnnotate, and even basic Python scripting can also boost task performance and open access to higher-tier work. These tools help structure tasks more efficiently, especially for complex datasets or custom labelling tasks.
Workers who consistently deliver high-quality results may receive direct invitations to long-term annotation projects or model evaluation work. Keeping detailed records of tasks completed, feedback received, and time invested is useful both for self-optimisation and for demonstrating professional reliability.